Towards the democratisation of open research information for scientometrics and science policy: the Campinas experience
The Barcelona Declaration represented a major step towards the adoption of open data for scientometrics and science policy. This blogpost discusses the importance of also moving towards democratising the access and use of these open resources, by sharing our experience from Campinas in Brazil.
A broad landscape of open research information systems
The landscape of open research information systems is broad. In addition to large (and global) open data sources like OpenAlex, OpenAIRE, or PubMed, we also have local or regional open data sources like SciELO, Redalyc or LaReferencia. In a previous blog post, we recently argued that such richness and diversity of data sources call for decentralised and federated perspectives in the use and adoption of open research information in scientometrics and science policy work.
Recent initiatives like the Barcelona Declaration or the Leiden Ranking Open Edition represent fundamental milestones towards the broader adoption of open research information systems in all types of meta-science activities, like monitoring, evaluation or management. These initiatives demonstrate how it is reasonable to “dream” that the future of scientometrics and science policy will be based on open, diverse and inclusive data sources, and accessible and usable by a much wider range of users than it has ever been (similar dreams were already expressed by Katz and Hicks in 1997 in their Desktop Scientometrics).
In the pursuit of such a dream, we have realised that a fundamental gap that still needs to be bridged is the question of how this large landscape of open research information systems could be best accessed and used by all relevant users, thus contributing to a more democratic access and benefit of the availability of these open data sources.
Towards democratisation of open research information systems
We have so much open data available, but researchers, managers and policy makers from several areas working on problems around science dynamics still face the problem of how to effectively access and work with them.
Just to illustrate the problem, let’s take OpenAlex as an example. OpenAlex offers its full database in an open snapshot, which opens the possibility for anyone to perform large scale analytics with the database. The problem is that the full snapshot of the OpenAlex database takes more than 470 GB of memory compressed and about 2 TB uncompressed. This alone represents a fundamental barrier for many users to download, process, work and benefit from the full database; the barrier being not only the infrastructural needs to process and work with the data (e.g. large computers, computer power, etc.), but also the technical skills that such an endeavour would require (e.g. programming languages, database management, etc.). OpenAlex is aware of this (and in all fairness, the efforts they have made to facilitate the access and use of the database are laudable!) and also offers an open API, which allows users to extract and work with smaller and more sizable datasets. However, the API may still pose technical limitations for many users, and its usefulness may be limited for some of the analytical questions that may be asked (e.g. for large scale researchers’ mobility analytics, or the calculation of field-normalised citation scores).
At this stage, some may argue “well, too bad, but this is the nature of things”. This does not need to be the case. Instead, we believe that in addition to openness and diversity of open research information systems, it is also important to democratise them by making them more accessible and usable to all relevant users who can reasonably participate and benefit from them, thus making truly possible an effective democratisation of the way we manage our scientific system.
In this blogpost we describe our experience in the InSySPo project, in Campinas, Brazil, of how concrete steps towards wider and easier access to open research information systems could be developed and implemented. We hope that our experience will encourage other users and stakeholders to engage and benefit from the availability of open research information data sources, and to seek for collective action regarding the sustainability, expansion, broad participation and use of these systems.
The Campinas experience: The InSySPo project
The InSySPo project (and team) started in 2020 at the University of Campinas (UNICAMP) in Brazil, with the aim of developing research in multidisciplinary fields that encompass economics, social sciences, scientometrics and innovation studies. Due to the ambitions and research demands of the project, particularly around the so-called Research Trajectory 4 (focused on Big Data for Assessing Innovation processes and Research Innovation Policy), we took the firm decision of using openly available data sources, including global data sources like OpenAlex, but also local ones like SciELO, among others.
After some attempts at investigating possible solutions with local hardware and servers, we finally opted to go with cloud-based infrastructures. This was motivated by a need to have a running operational infrastructure in a relatively short time and to rapidly provide access to all the members of the project, as well as collaborators and associated partners.
The GBQ infrastructure
Inspired by the solutions already used in small and medium-sized companies as well as in the data industry as a whole, and considering our limited budget of BRL 500,000 Reais (about EUR 90,000) for developing the project data infrastructure, we opted for using a general purpose cloud computing provider. In our case we opted for Google Big Query (GBQ - Figure 1 shows a screenshot of the main InSySPo GBQ interface), although other options like Amazon, Microsoft and Alibaba were also explored.
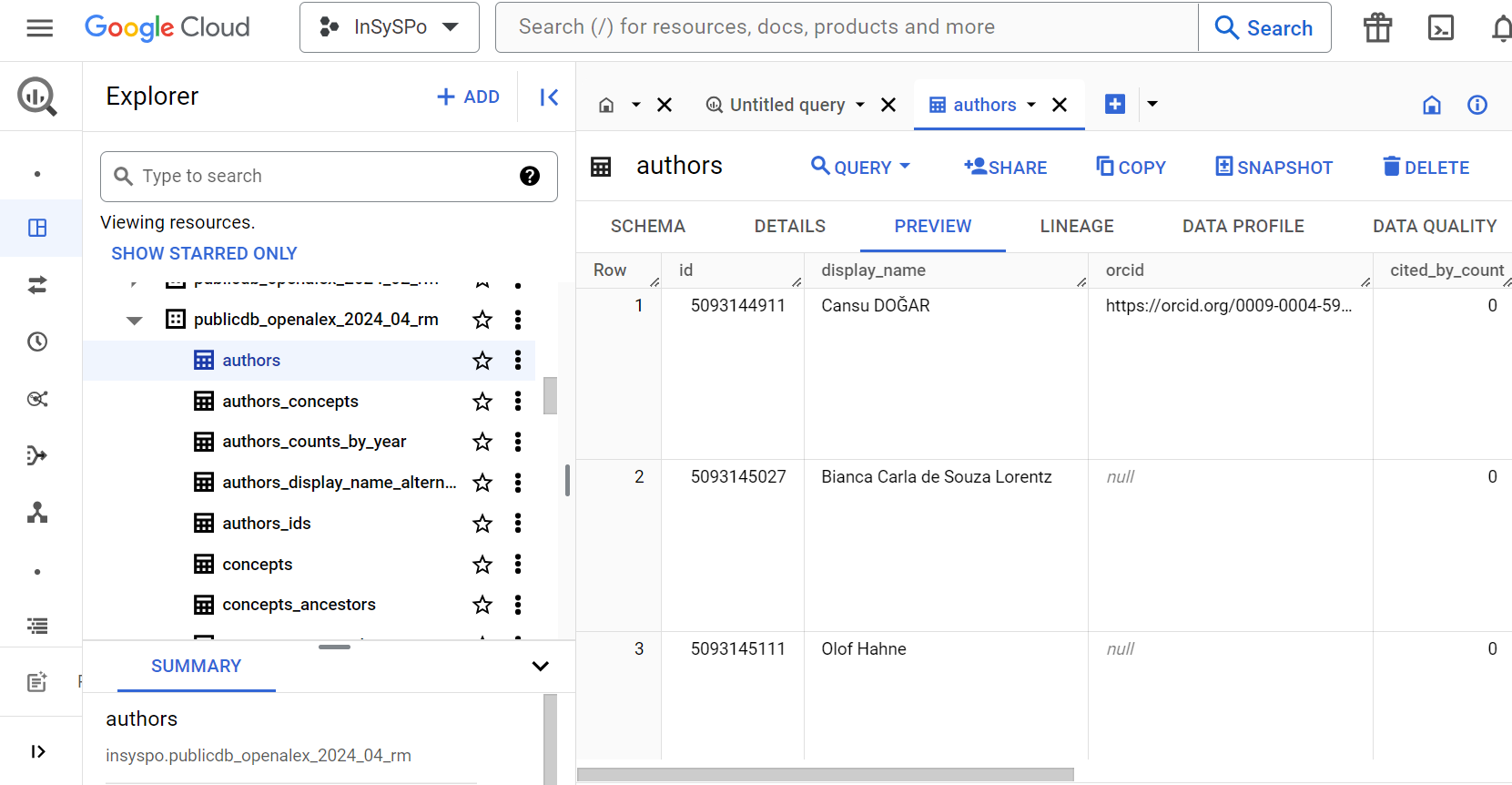
The advantages of choosing a cloud computing solution can be summarised as:
- no need for physical infrastructure, which would have taken time and substantial resources;
- simplicity of including and providing access to users;
- computational environments for users to work and analyse the data.
The main disadvantage however is that we are using proprietary technology (i.e. Google), and that there are still computational costs that need to be considered. However, the overall costs of the use of the infrastructure have been manageable within our budget restrictions all throughout the project’s running time.
In less than a year, the users of the project already had access to data sources like OpenAlex, SciELO or PATSTAT, to name a few.
Our open data sources and public datasets in GBQ
The choice of the GBQ allowed us to provide ‘public’ access to all our open data sources. This means that all the open databases we have already created could theoretically be used by everyone with a Google account. That way, databases developed by our group can be used by others at their own expense, and vice versa (e.g. users could also combine the GBQ data of the Leiden Ranking Open Edition, or Dimensions’ public versions). Intuitively, this also makes feasible a potential federation of different data sources on a single platform, contributed and used by multiple actors.
Just by accessing the project’s profile on BigQuery, anyone can see and query the public datasets we have created (see Table 1 for a full list) at their own needs and pay for their own querying. Approximately, 1TB of queries costs US$5, an amount which can pay for a few small projects. Further estimations can be made using the pricing calculator.
Data source |
Latest version |
Implemented |
Description |
04/2024 |
Fully relational model |
Comprehensive database of scholarly metadata for publications. |
|
04/2024 |
Fully relational model |
Metadata for Scientific Electronic Library Online, comprising Latin American, Caribbean, South Africa. |
|
01/2024 |
Relational with nested fields |
Metadata for mostly European research projects. |
|
10/2022 |
JSON table |
Researchers persistent identifier and metadata. |
|
10/2022 |
JSON table |
Organisation that registers digital object identifiers for publications. |
|
06/2022 |
Fully relational model |
Brazilian database of intellectual property data. |
|
12/2022 |
Fully relational model |
Metadata about Brazilian postgraduate programmes. |
Table 1. List of public data sources available in the GBQ InSySPo infrastructure. They can be accessed at console.cloud.google.com/bigquery?project=insyspo and the code for their implementation and analysis is in repositories at github.com/insyspo.
It is important to note that databases in GBQ can be implemented with different degrees of relational implementation. Thus, for example, OpenAlex and SciELO have been implemented in full relational models, while OpenAIRE is only partially implemented in a relational model, still having some nested fields in JSON format, or ORCID which is only in JSON format. Those decisions are related to the frequency of use of the databases as a trade off between cost and performance.
How to work with it?
We now have about 200 users that are part of the project and have access not only to the public but also non-public datasets related to their activities and can use the system. The cost of our infrastructure is about 300 US dollars a month. Users work directly on the GBQ interface, particularly to run SQL queries, or using Google Colaboratory (Colab) (See Figure 2 for an example). For users with large computing needs, we also grant command-line access to specific assets, allowing them to run queries and execute virtual machine tasks on the preferred platform such as VS Code or DBeaver by means of access to the Google Cloud command line interface (GCP CLI). Google Cloud Platform manages the privileges for users on a fine-grained level. The platform uses the concept of roles to define users that are common collections of privileges and it is possible to create roles for your own needs. In our case we add new users by giving them basic access, and then, for every specific asset such as datasets, storage buckets, virtual machines, we provide specific owner or editor access on the asset level.
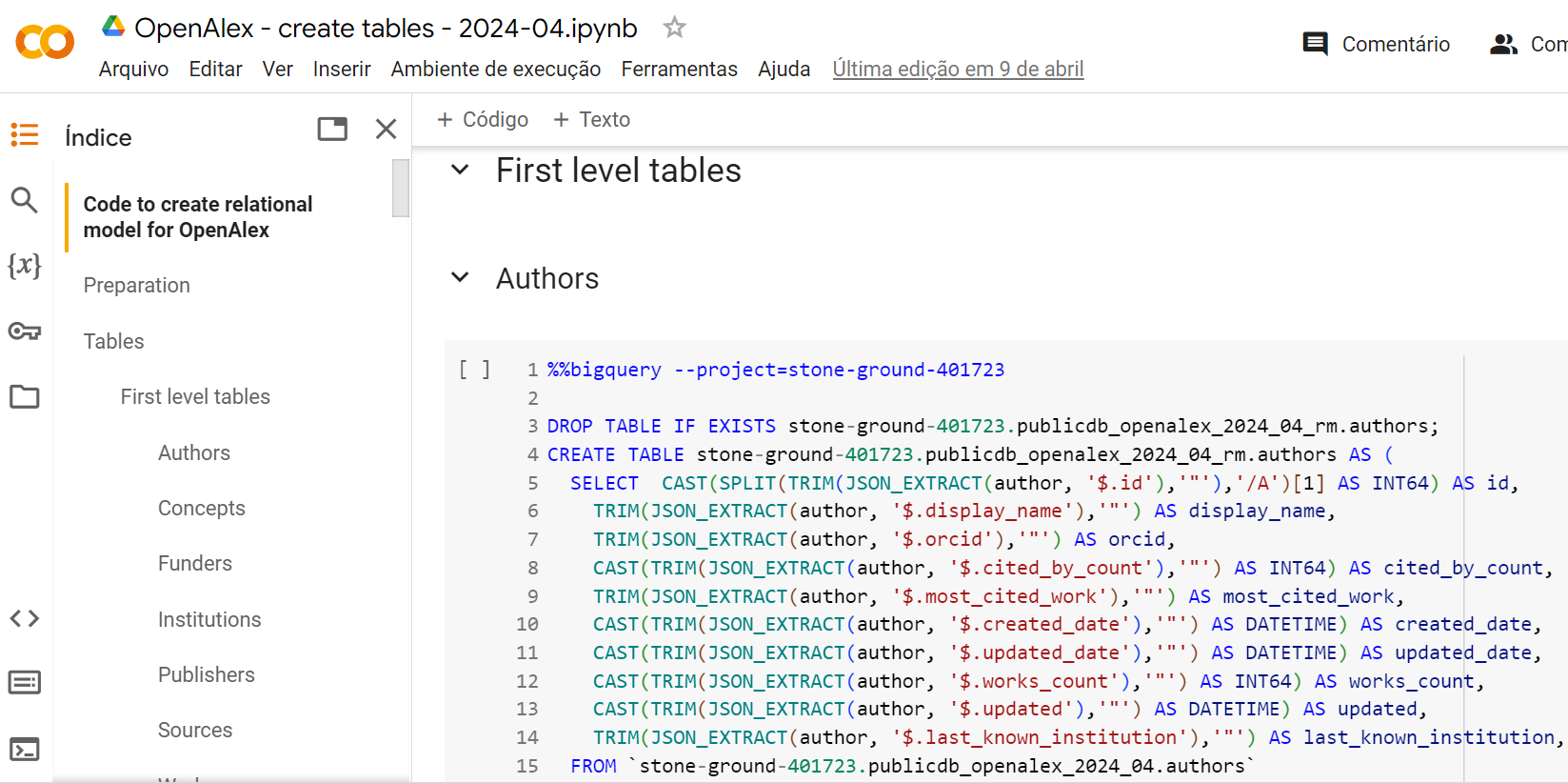
Colab can be made into a centralised mix of code and documentation that follows a scholarly work or used standalone. In practical terms, this makes it possible to share the full script of a paper, including data collection, analysis and results in one script (see an example here). In other words, it is a straightforward way of making scientometrics work not only easier and more exchangeable, but also more transparent.
The road ahead and open questions
Our experience in Campinas demonstrated that it is indeed possible to bridge the gap between available open research data and a wider range of users, simply by creating and facilitating working spaces where users with different degrees of expertise, technical skills and analytical questions, can directly query and interact with the data. Our experience demonstrated that it is possible for anyone to 1) access and work with the public datasets we have made available (with the only constraint of having to pay for their own querying computing power); and 2) to develop a similar infrastructure, with similar approaches and diversity of sources as we have done it in Campinas (see ‘Do It Yourself’ below).
Obviously, our experience is just a proof of concept of how a more ideal democratised open research information system could take shape. There are still important aspects that will require further attention in the near future to achieve the dream of an open, diverse and more participatory landscape of open research information systems:
- More work is needed in the processing, interconnection and federation of multiple data sources. As discussed before, and particularly in the context of Global South research, it is important to maintain and develop the richness of a multiplicity of data sources and perspectives. To achieve this, it is paramount that existing data sources can be easily interconnected, thus requiring extensive work of matching and interconnecting the different elements of the data sources (e.g. publication identifiers, researchers, organisations, etc.).
- In the process of democratising the use of open research information systems, training and capacity building for users is paramount. Providing users with the basic technical and analytical skills to work and benefit from the data should be seen as a key element for an effective, ethical and responsible use of open research information. Thus, the development of tutorials, courses, educational material, best practices, etc. should be central in the future agenda of this democratisation.
- We cannot live completely apart from the geopolitical tensions and we understand that Google Cloud infrastructure is not easily accessible in a few countries, for example in China. However, the underlying technologies used by cloud providers are used everywhere. A very similar framework could be set up in Alibaba Cloud services, for example. A cross integration based on storage systems can even be tried. This is not our most pressing need at the moment, but we invite anyone interested in working with us in this direction.
- Finally, there are important sustainability and collective questions that need to be addressed in the near future. How could an infrastructure like this be sustained? Could we go beyond Google and consider other non-proprietary solutions that could allow users to engage and work with the same large data sets and simplicity we have experienced? How could the work and costs be distributed and shared among many different partners? Plans for collaborative and more participatory approaches are needed, and initiatives like the Barcelona Declaration represent a decisive step in this direction. Cooperative efforts in sharing costs, platforms, data contributions (e.g. with new data sources, algorithms, matching approaches, etc.) and provision of training and capacity building would be stepping stones towards a sustainable landscape of open and diverse research information, ‘democratically’ useful for all users and communities interested in open research information.
Do It Yourself
- If you plan to set up a similar system on the same cloud provider as we did, you should first create an account and activate a billing account on Google Cloud Platform
- After that you can start using the several tools, for example use Google Cloud Storage to keep your datasets
- Use BigQuery as an SQL-based data warehouse
- Instead of creating your own infrastructure, you can directly use the different public datasets we created
- Use notebooks with Google Colaboratory that access datasets on other parts of the platform
- We also have provided training on the combination of tools and how to use SQL for querying the datasets via YoSigoPublicando:
- We have public code for the implementation, organisation and use of several datasets available on Github: https://github.com/insyspo
If you have any questions or suggestions on how to contribute and expand the ideas presented in this blogpost, do not hesitate in contacting the authors of this blog (Alysson Mazoni / Rodrigo Costas)!
Header image: Pixabay
DOI: 10.59350/eqmfk-82y98 (export/download/cite this blog post)
0 Comments
Add a comment